One can have little doubt that in the third decade of the 21st century, digital technologies have made an enormous impact on virtually every aspect of human endeavor, including on the healthcare sector. Ingenious software solutions, for example, are already transforming previously laborious but necessary tasks. These range from care administration remedies that auto-update themselves with real-time new data on patients to automated billing and coding solutions, which help improve healthcare delivery and patient outcomes.
Yet, one phenomenon that has silently accompanied this digital revolution has now reached such colossal proportions that it might be considered both a blessing and a curse. The phenomenon can be summarized in two words: digital data. Mountains of it. Every digitally-executed task generates its own footprint of digital data, and there are countless numbers of digitally-executed tasks going on around the world every second of every day.
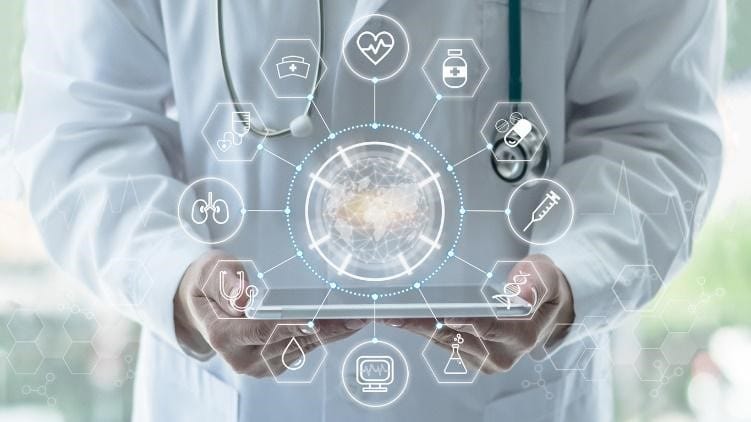
The “curse” side of the equation resides in the fact that it’s estimated to generate 44 zettabytes (that’s 44 billion terabytes) of digital data every day, and this continues to increase. These quantities are well beyond the mere human powers of comprehension and calculus.
The “blessing” side comes in the form of one of the many offshoots of human ingenuity: the capacity to devise ingenious digital solutions to the mind-confounding galaxies of data we’re continually immersed in. These solutions capture it, organize it and help generate intelligible insights that may lead to much more informed real-world interventions, such as in healthcare.
In what follows, we’ll explore why the analysis of data has become of pivotal importance in healthcare, including how it improves the quality of practice, as well as the clinical insights of healthcare professionals, how it can detect easily-missed anomalies in complex scans, how it facilitates more personalized treatment and how it enables faster and more accurate diagnoses.
Data analysis in healthcare: The four key types
One way of thinking about the matter at hand is to couch it in the form of a motto: “out of quantity, data analytics aims to yield quality”.
This quality has the effect of extending clinical perception and of aiding better decision-making about patient care and healthcare delivery to communities. Yet, given that we are assessing unimaginably vast quantities of digital data, how does one begin trying to sort it?
In healthcare, those mountain ranges of data are subject to four broad kinds of data analysis, including:
- Descriptive analysis: the analysis of events or conditions that have already occurred. For example, a virus can be assessed for its contagiousness by examining data collected from previous positive test results in a specific population.
- Diagnostic analysis: the analysis of data pertaining to the cause of a clinical condition, such as diabetes or rheumatoid arthritis, or an ‘event’, such as an injury, heart attack or a stroke, in order to make an accurate diagnosis from presenting symptoms.
- Predictive analytics: the analysis of historical data and previous trends in relation to a specific condition, or treatment/management options in order to make informed projections about likely future developments. An example might be the likely spread of a seasonal disease such as influenza.
- Prescriptive analytics: The use of collected data to inform decisions in relation to issues such as individual patient treatment options or the delivery and management of healthcare services, with the aim of achieving optimal outcomes. Such data may be used to assess an individual patient’s risk of developing conditions in the future based upon their previous medical history, and to craft effective preventative treatment strategies to minimize that risk. However, as noted in a 2023 article in Forbes, genomic data can now be used to group patients into risk profiles based on biomarker discovery, prognosis and disease subtyping. In turn, this helps clinicians to devise personalized, targeted treatments for them and facilitate improved long-term outcomes.
How data analytics are used in healthcare
Practitioner development
Clinicians can learn a great deal from their patients. Sometimes this is related to their knowledge and skill in assessment, diagnosis and treatment – but at other times, it’s connected to how well they listen to their patients. Listening in this respect isn’t simply confined to what patients vocalize, but involves a kind of “listening with one’s eyes”, which involves reading patients’ facial expressions, bodily gestures, level of apparent anxiety, and so on, all of which are forms of communication.
An important study on physician empathy headed by Dr Helen Reiss in 2007, for example, found that many clinicians were inclined to prioritize objective scientific facts about a patient’s condition over the latter’s own direct experience of their symptoms. Her study invited patients to rate their evaluation of a doctors’ degree of empathy on a scale. When the data was collected, half of the physicians in the study were given training on how to include empathic listening in their clinical assessments, while the other half were not.
Upon a second empathy rating questionnaire, it was clear that doctors who received the training were ranked more highly by patients than the control group.
As clinician empathy has been shown to be strongly correlated with improved patient safety and reduced malpractice claims, this is a valuable, key enhancement of any practicing physician’s skills repertoire.
Detecting clinical abnormalities/anomalies in scans
Expertly-honed medical knowledge and perception will always be indispensable in clinical practice; however, these abilities can be significantly enhanced, courtesy of machine learning algorithms. The latter can analyze data considerably faster and more efficiently than even the most highly-trained and knowledgeable human beings are able to do.
An example is the “VoxelMorph” algorithm developed by researchers at the Massachusetts Institute of Technology in 2018. Deploying a machine learning approach powered by a “Convolutional Neural Network”, which was devised to assist in image processing, the algorithm was able to detect differences between 3D medical images such as MRI scans. Essentially, it learns data from a patient’s previous MRI and then compares it with the data contained in a subsequent scan. In so doing, it’s able to pinpoint differences between scans 1,000 times faster than an expertly-trained human clinician can!
The clinician’s knowledge, of course, remains of pivotal importance, not least of all in knowing what treatment(s) to proceed with to secure the best clinical outcome for a patient. However, the speed of the machine learning algorithm in this application not only saves time, but may also save lives as if a change is overlooked by a human eye, an algorithm like this will pick it up.
In other words, healthcare analytics, when deployed and understood correctly, are able to deliver more personalized patient care and facilitate faster diagnoses, both of which play a part in saving lives by allowing effective treatments to be initiated for a potentially serious condition before it has advanced to an untreatable degree.
Healthcare data analytics and population health management
To zoom out for a moment from the world of individual face-to-face patient treatment and care, healthcare data analytics is proving immensely valuable in promoting improved health outcomes at the level of populations.
Population health management refers to the work of improving the healthcare outcomes for a designated group of patients by coordinating their care in a better way. This is a process which includes improving their engagement with the treatments that will benefit them.
But what, exactly, does this work consist of in the age of digital data? Essentially, by engaging data scientists to craft predictive artificial intelligence (AI) health outcomes, which in turn assists healthcare organizations in managing initiatives informed by these models for selected populations who are known to be in need of health care.
An example would be to target a population known to be vulnerable for active outreach to improve their engagement with effective treatments and help them with relapse prevention strategies. The patients thereby benefit from improved engagement and better clinical outcomes, and healthcare organizations benefit by maximizing their efficiency.
Other applications of healthcare analytics
Assessing co-morbidities
If the great 17th century scholar and poet John Donne was right in his luminous observation that “No man is an island”, then modern medicine might productively adapt this for disease – no illness is an island. Just as Donne was emphasizing that, even when alone, a human being is always connected with other people — if not in immediate reality, then in heart and mind — it is equally true that modern medicine has learned that no illness is an island unto itself.
Clinicians have learned to be vigilant about the presence and effects of co-morbidities, especially for those with multiple effects such as type 2 diabetes. Data analytics looks set to play a new and important role in discerning the impact of these coexisting conditions, predicting which patients are more at risk of developing serious complications or of developing life-threating conditions such as sepsis upon a prolonged hospitalization. These risk statistics and forecasts have the potential to help doctors craft intricate, personalized treatment packages that slow disease progression.
Improving patient attendance at pre-arranged appointments
It’s a sad fact of life that non-attendance at pre-scheduled appointments produces plenty of ill-effects. It wastes money and time as an unused appointment is simply “dead time” that could have been better used for patient assessment and treatment, and paying doctors, radiographers or MRI scan technicians to twiddle their thumbs during missed appointments is an unconscionable waste of resources.
A 2020 study published in the American Journal of Roentgenology, however, found that a combination of AI, predictive analytics and a system of simple reminders for patients with pre-scheduled MRI appointments resulted in a 17% rise in attendance.
Gaining the know-how for a career in healthcare data analytics
As we have seen in the preceding paragraphs, data analytics in healthcare benefits not only clinicians and their patients, but entire healthcare organizations.
Just as technology is facilitating the rise of a new kind of clinician, it’s also facilitating demand for a new kind of healthcare administrator. The fact is that with the advent of the age of big data, aptitudes in data analytics have become increasingly indispensable for aspiring healthcare executives. Thankfully, for those sharing such aspirations, getting the proper credentials with the advanced qualifications needed to fulfill such expert executive roles has never been more accessible.
For example, due to rising demand, a well-established traditional center of higher academic excellence such as the University of Ottawa’s Telfer School of Management is now offering a unique online master degree program that specifically intersects both healthcare and business management. The Telfer Executive Master of Health Administration (EMHA) equips its graduates with rigorous knowledge of health systems and management fundamentals, as informed by data, technology and policy know-how.
Familiarity with the types and methods of data analysis has effectively become an essential prerequisite for modern healthcare executives, just as it has for clinicians. The ability to transform a seemingly dizzying mess of numbers and words into intelligible and relevant information has never been so sought-after as it is today. As the healthcare industry has embraced digital technologies with gusto, so too has the influx of digital data thereby generated reached truly vast proportions. Lacking data analytic expertise in a context such as this is almost a recipe for failure. If the data exists, which it certainly does, then it cries out to be analyzed and rendered intelligible so that it can be harnessed and used pragmatically for beneficial ends.
In short, big data and the expertise required to understand it and use it has entered not only the clinical and operational side of healthcare but the administrative and financial side as well. Here it’s proving to be every bit as crucial to the provision of effective service delivery and, ultimately, saving patients’ lives, as it is in the former.
Given that healthcare data is among the most complicated data generated by any industry, whether it’s generated by recording patients’ vital signs in real-time, analyzing MRI scans for anomalies or updating secure electronic healthcare records, alongside numerous other applications, it must be captured, safely stored and properly analyzed if it is to have value. This is where executives possessing rigorous data analytics knowledge alongside their knowledge of healthcare management really come into their own.